The RBI’s annual report for 2023-24 had a positive outlook on the financial inclusion (FI) progress and achievement of priority sector lending targets (see Table 1).
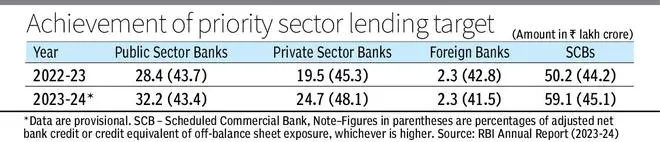
Credit access, utilisation, and service quality are critical enablers in reducing poverty and boosting priority sector activities.
One of the priority sectors is the agriculture sector.
The FI index has moved up from 43.4 in 2017 to 60.1 in 2023, with ₹21.55-lakh crore of agricultural credit disbursed.
However, how much agri-lending was organic, inorganic, or forced is not clear.
Second, RBI reported that the number of operative Kisan Credit Cards (KCCs) increased by 5.4 per cent in 2023-24 from 2022-23, with the outstanding amount up 10.9 per cent.
Nonetheless, the operative KCC accounts do not include non-performing asset accounts and less than 50 per cent of farmer population (7.36 crores) have availed itself of KCCs as a single-window facility for working capital and investment agricultural credit. In other words, more than 50 per cent of farmers have not utilised formal financial services.
What constrains farmers’ access institutional credit or formal finance? The absence of robust borrower data increases search costs and compels mainstream lenders to rely on credit scoring models for loan applicant evaluation, processing, and sanction.
The extant credit scoring model classifies applicants into the solvent (likely to repay without credit default) and delinquents (prone to non-repayment). Banks differentiate between “good” and “bad” credit risks to streamline financing decisions and minimise portfolio (default) risks.
Credit scoring models depend on the borrower’s financial history, including the length of credit history, amount of credit availed, and credit utilisation ratio, which weigh heavily on credit scores.
In contrast, low-income individuals such as small and marginal farmers typically do not have the financial or credit history that credit bureaus require to determine credit scores. This lack of data presents an uphill task in deciding farmers’ creditworthiness (USAID-DFS, 2016).
So, how can an alternative scoring model push institutional finance? The World Bank Group emphasised using alternative data sources to generate credit scores for potential applicants and analyse farmers’ personal, property, and liability risks. It involves sourcing various types of alternative data and machine learning algorithms to train and test these data to predict the likelihood of credit default.
Alternative Scoring Model
First, alternative indicators are of two types — non-transactional and transactional.
Non-transactional indicators include farmers’ demographic, psychometric, and digital footprints, while transactional indicators include utility payments, e-commerce or platform usage, and mobile usage.
Second, attributes of non-transactional and transactional indicators can be modelled to diagnose and predict the behaviour of unbanked farmers, and classify them into prospective or solvent and non-prospective or delinquent borrowers. Machine learning models are effective in classifying user groups.
Third, a list of predictors must be identified to determine the alternative scoring factors, such as unbanked farmers’ fraud score through farmer classification, interaction score based on farmer interaction with fellow farmers and agri-value chain actors in the marketplace, and revenue score based on their farming activities. The sensitivity, specificity, and accuracy of these score-generating models must be tested and validated.
Fourth, alternative scoring models can serve as a surrogate measure of credit analysis model encompassing character, capacity, capital, condition, and collateral.
To map the non-financial data, merchants, data curators, and technology companies must be roped in to provide legitimate alternative indicators and share application programming interfaces to decode data-driven models for bankers’ interpretation and action.
Tyagi is a doctoral scholar, and Kapoor and Dey teach at IIM Lucknow. Views expressed are personal.
Comments
Comments have to be in English, and in full sentences. They cannot be abusive or personal. Please abide by our community guidelines for posting your comments.
We have migrated to a new commenting platform. If you are already a registered user of TheHindu Businessline and logged in, you may continue to engage with our articles. If you do not have an account please register and login to post comments. Users can access their older comments by logging into their accounts on Vuukle.